Exclusive Interview:
Doug Shannon, Automation and AI practitioner and Gartner Peer Community Ambassador
Connect with Doug Shannon on LinkedIn
speaks with Independent Tech Journalist Pat Brans
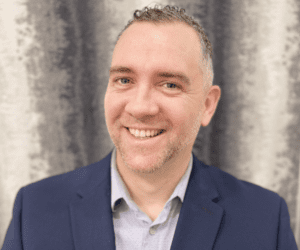
How Automation helps enterprises manage the data they need for AI
Most of the IT leaders who began experimenting with generative AI sometime in the last 12 months have learned that successful implementation relies on robust methods for collecting and managing data.
“GenAI is still very hard to implement in the enterprise,” says Doug Shannon, Automation and AI practitioner and Gartner Peer Community Ambassador.
“Most of the organisations who started early found out very quickly how expensive it can get.”
IT leaders became painfully aware of how difficult it is to predict and control the cost of GenAI. After paying for initial implementation, they are often surprised by the ongoing costs. Enterprises are charged by the number of words returned in response to a prompt. With hundreds or thousands of employees asking questions on a range of topics, generating wordy response, there’s no telling what will appear on the bill. On top of those expenses, enterprise also pay for daily use of software platforms, such as Copilot.
“If you’re just using GenAI to help with meetings or email, it might not justify the cost… The trick is to find the use cases that make it worthwhile.”
The biggest use case for the time being is around making knowledge available to enterprise users, says Shannon. IT leaders might build a knowledge base from all the information in the company to boost productivity. Another way of providing access to knowledge is to use retrieval augmented generative models (RAG models), which allow people to “talk to their data.”
It’s All About the Data
In the end, though, nobody will achieve the ROI they need unless they collect the right data and manage it in a robust manner. Because most companies need an AI that knows something about their business, they have to go beyond a general language model and undergo subsequent phases of training where the algorithm learns data specific to the company and industry.
“You have this foundational model that you get from one of the big platform players. On the back end, you have these things called data blobs in Azure (or something equivalent in other solutions) that allow you to take stores of your enterprise data and chunk it.
The blobs get indexed and then they get updated whenever new data is added to these areas. It’s up to the enterprise to put the right data in there in the first place.”
Much of the existing knowledge base is stored in legacy applications. In large companies, hundreds of thousands of users have put information into those applications at different times and in different contexts, depending on their job roles. As for small and medium sized companies with less data of their own, sometimes third party data is used to complement what they have in-house—and some of the more forward-looking companies are even experimenting with ways of synthesising data to fill in the gaps.
Be Mindful of Regulations
Once information is collected, it needs to be categorised, filtered, and normalised before it can be used to train an AI. Enterprises need to be mindful of regulations in one or more parts of the world, paying particular attention to confidentiality and rules like GDPR. Above all, IT departments need to keep records of data trails for potential audits.
According to Shannon, to ensure responsible use of GenAI, data collection and management should be built in a way that allows enterprises to identify three things. He calls them the chain of thought, the chain of reasoning, and the chain of custody.
Shannon defines the chain of thought as the set of information that leads an AI to a given result. For example, if a user prompts a tool to find out how many products were sold to young adults in London, the answer might come from a combination of documents, including sales figures and customer profiles. The chain of reasoning, says Shannon, is the context in which the question was asked. For example, if somebody from customer support asks a question, the AI gives a response that assumes the goal is to help a customer. And Shannon describes the chain of custody as a record of where data has been and who changed it. For example, information might be collected from a marketing event, updated by a salesperson during the first presentation, and subsequently modified by pre-sales technical consultants.
Moreover, says Shannon, to minimise hallucinations, GenAI should be trained on up-to-date information sources to “ground” the data.
“When you have good grounding, your hallucinations go down to something like 2% or less, which is a massive improvement in most cases,” he says.
How data is categorised, filtered, and normalised depends on whether it’s structured or unstructured. Structured data is much easier to handle because it conforms to a known format. However, unstructured data is far more complicated to process, because it’s literally anything and everything all at once. IT departments need tools to break it down and normalise it so the context and ontology of the data can be understood for use with AI.
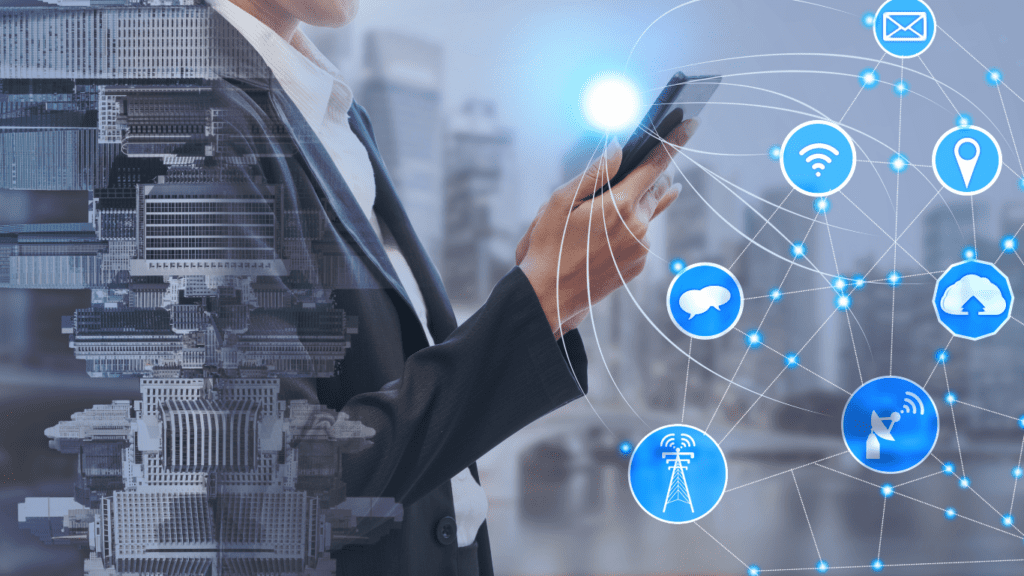
Without Data Pipeline Orchestration there is No AI
According to Shannon, role-based access controls (RBAC) is also a big pain point for GenAI. “Even with some of the larger companies like Microsoft, when you pull in information from your data pipeline, it strips out all of your RBAC,” he says. “So you no longer know where the information came from and who had access to it.”
Data pipeline management is essential for making sure you know where data has been, where it’s stored, and how it’s governed. “You know when, where, and why the data is needed—and you need to know the chain of custody—including access controls,” says Shannon.
Data pipelines have to be orchestrated to collect relevant and timely information from the right data stores. Forward-looking companies use a service orchestration platform to centrally configure and monitor critical data pipelines, such as the ones needed to train AI.
A service orchestration platform consists of a central administration point, which is the heart of the tool, and a set of agents that reach out into different types of cloud or on-premises systems. This arrangement allow IT departments to automate and visualise processes, workflows, and data pipelines involving disparate systems running both inside and outside their organisations.
Your Next Steps
“Organisations need to maintain tight control over their training data,” says Martin Hulbert, CTO of Ignite Technology. “Only those who get that right benefit from the power of AI.”.
Wherever you are on your automation journey, Ignite Technology’s Broadcom expertise will help you get the most from your automation strategy and maximise efficiency throughout your organisation. Through our Broadcom Expert Advantage Partner status, Ignite provide tailored services, ensuring that we don’t just deliver technology; it delivers change, bespoke to the unique needs of clients through our consultation, implementation, and support expertise.
Get in touch with our team today for more information on how you can empower your AI initiatives through Automation.